AI Music Ensemble Technology
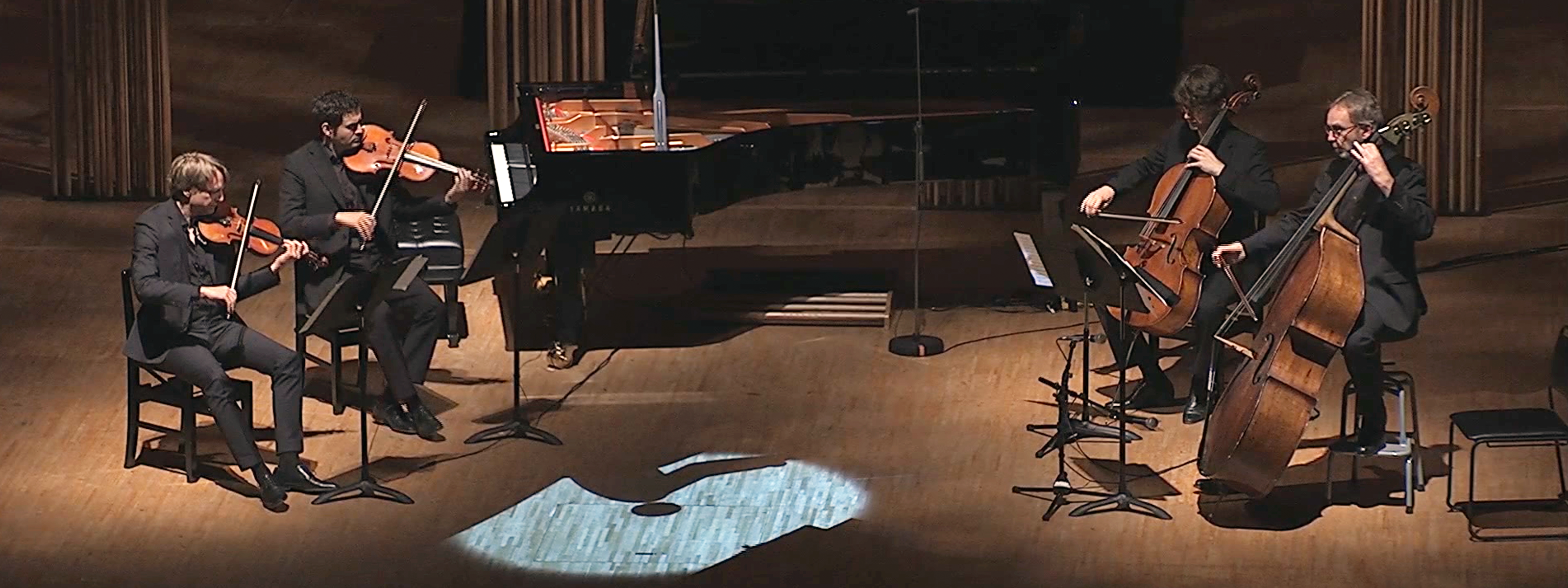
AI musician that listens and synchronizes with the player
This AI technology allows a machine musician to play with human musicians in a music ensemble. It analyzes human performance in real-time to generate a synchronized musical performance that matches the human player’s musical expression.
The AI compares the sound of the human performance with the music score data of the piece being played, and analyzes where in the score the human performance is currently playing, at what speed, and with what kind of musical expression. In response, it can generate a performance that matches the timing of the human performance by predicting the performance a little further ahead.
AI can analyze various input sources, including a piano, a musical ensemble of acoustic instruments like violins and flutes, multiple players, and orchestras. The analysis results of the human performance can be used to accompany the human players on a piano or to control various devices such as lighting and video for a performance.
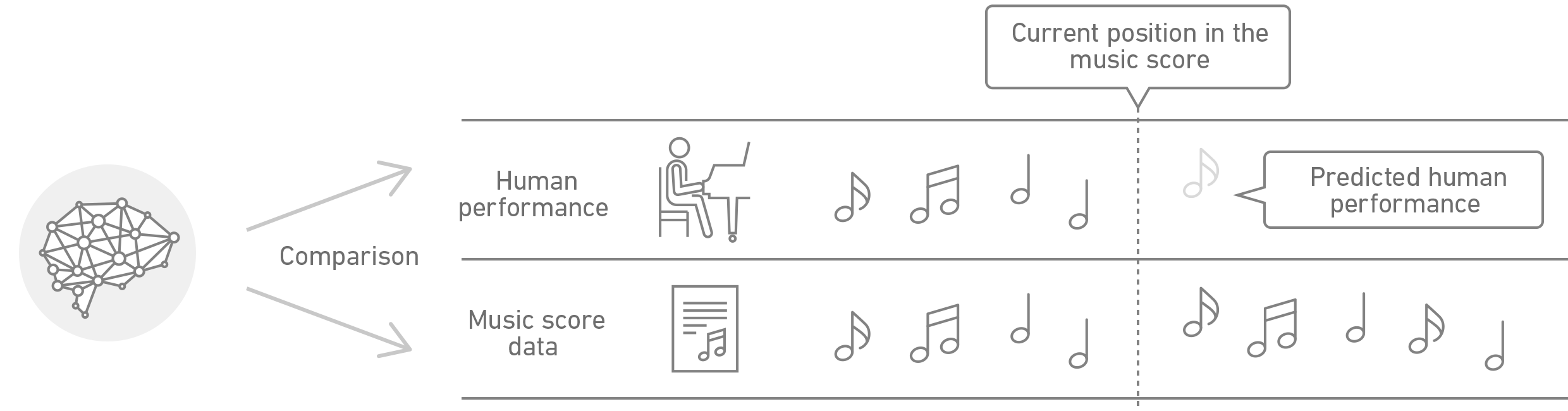
How it Works
The AI analyzes human performance from four perspectives.
1. Multimodal Analysis of Sound and Movement
The AI uses microphones to listen to the sound being played and analyzes various information about the music performance. It can also capture the anticipatory movements of the performer with a camera to better predict the timing of the music performance.

2. Inference of Timing Fluctuations and Expression that is Robust Against Mistakes
AI can infer the tempo and expression of a human performer by comparing the music score with the human performance.
Since human performance includes intentional and unintentional performance fluctuations, such as tempo fluctuation and errors, it is necessary to compare how humans vary their performance when playing from a music score. Therefore, AI learns typical mistakes and deviations from the score in human performances by learning how the music score maps to the human performance. This will enable the system to act appropriately when a performer stumbles or makes a mistake.
AI can also recognize expressions based on articulation and dynamics, such as “lively” or “quiet,” by learning the various performance expressions of a piece of music in advance. Thus, it can generate accompaniment according to the overall expression of the human performance.
In addition, if the AI is trained specifically for a particular individual’s performance, it will be able to learn that person’s unique tempo fluctuations and tendency to make mistakes.
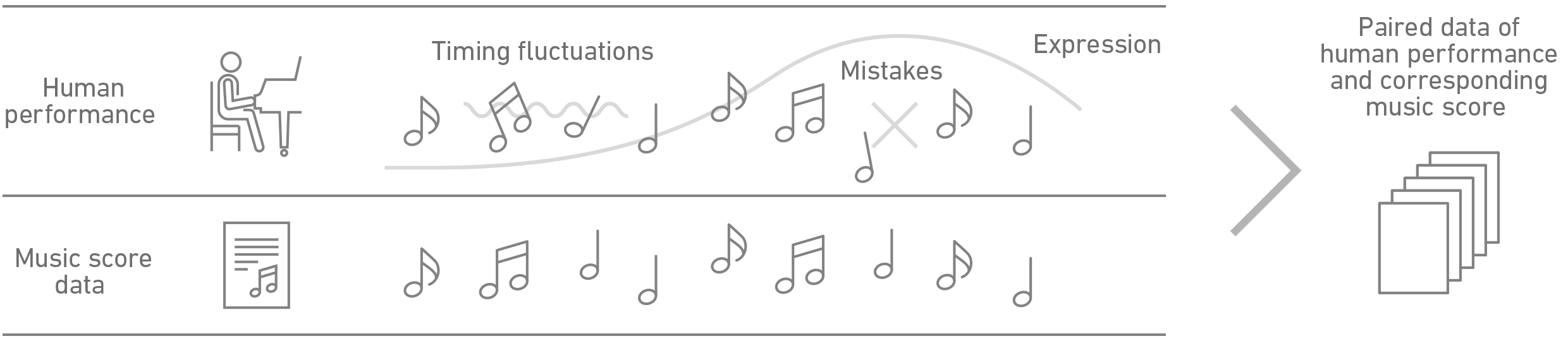
3. Timing Adjustment
Coordination is at the heart of a music ensemble. When listening to a human ensemble, it may sound as if everyone controls the tempo with an equal contribution. In reality, some musical parts have more control over others, and the degree of control changes according to the musical context of each part. This kind of subtle delegation of control of timing is essential for coordination in a music ensemble that fits the musical context.
The AI learns such timing adjustments by analyzing how humans perform with each other and the corresponding score data. By understanding the tendencies of the human performers, such as when they concede to the other performers and when they take the lead in the performance, it will be able to correct the timing musically according to the context of the score.
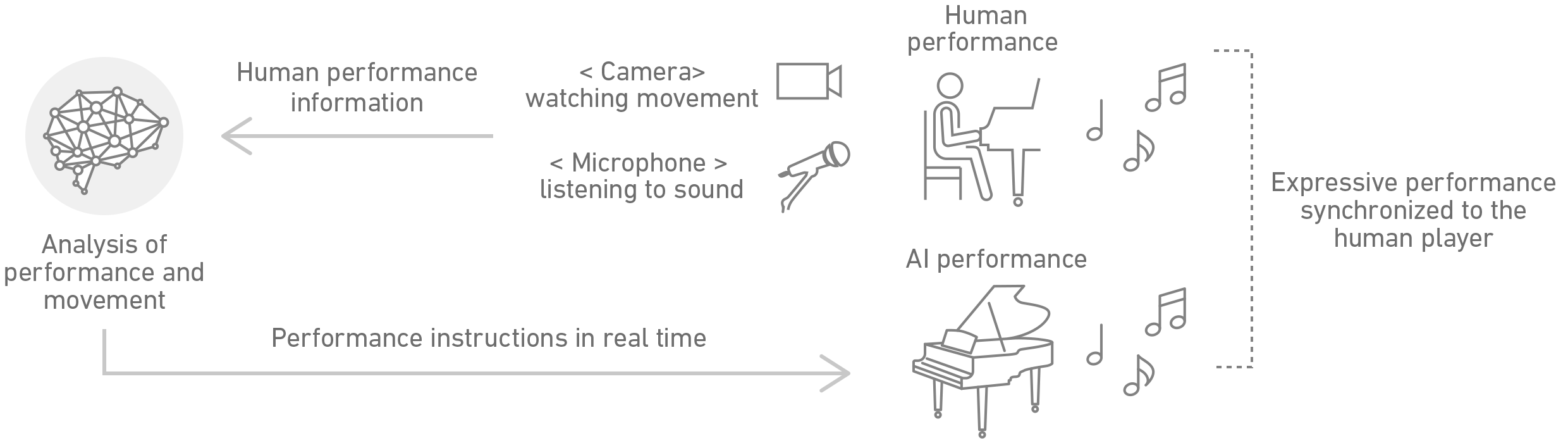
Application Examples
Projects Using This Technology: Project Sekai Piano
An “AI Music Ensemble Technology” was used for the “Project Sekai Piano” installation on exhibit from Friday, March 26 to Sunday, June 27, 2021. The “Project Sekai Piano” project allows virtual singers Hatsune Miku and Hoshino Ichika (CV: Noguchi Ruriko) to sing along with your performance of “Senbonzakura” (lyrics and music by Kurousa P) and “Aokukakero!” (lyrics and music by Marasy).
Hatsune Miku and Hoshino Issaka sing along with your performance! (Japanese)
Project Using this Technology: Otomai-no-Shirabe – Transcending Time and Space
On Thursday, May 19, 2016, we used “AI Music Ensemble Technology” to reproduce the performance of legendary pianist Sviatoslav Richter at the concert “Otomai-no-Shirabe: Transcendental Time and Space” held at the Sogakudo of Tokyo University of the Arts. On Thursday, May 19, 2016, we used “AI Music Ensemble Technology” to reproduce the performance of legendary pianist Sviatoslav Richter at the concert “Otomai-no-Shirabe: Transcendental Time and Space” held at the Sogakudo of Tokyo University of the Arts.
This made it possible for the Berliner Philharmoniker’s Scharoun Ensemble to perform with legendary pianist Sviatoslav Richter on a stage transcending time and space.
Concert at Tokyo University of the Arts / Otomai-no-shirabe (Japanese)
Related Items
Technical Exhibits
- Tokyo Music Evening Yube / Violin Recital by Tatsuki Narita
- Project Sekai Piano / Hatsune Miku and Hoshino Ichika sings with your piano performance!
- Ars Electronica Festival 2019 / Dear Glenn
- SALAD Music Festival 2019 / Music conducting simulation of Tokyo Metrop olitan Symphony Orchestra
- Daredemo Piano Exhibit
- SALAD Music Festival 2018 / You can improvise on Pachelbel’s Canon with Tokyo Metropolitan Symphony Orchestra and AI
- South by Southwest 2018 / Duet with YOO
- Concert at Tokyo University of the Arts / Mai-Hitenyu
- DIGITAL CONTENT EXPO 2017 / Music Ensemble of the Future
- Concert at Tokyo University of the Arts / Otomai-no-shirabe
- Blue Line Tokyo Creative Exhibition
- Nikoniko Chokaigi